Do more research,
for less wrangling
Transform messy data into structured schemas using readable, auditable methods. Perform schema-to-schema crosswalks for interoperability and data reuse.
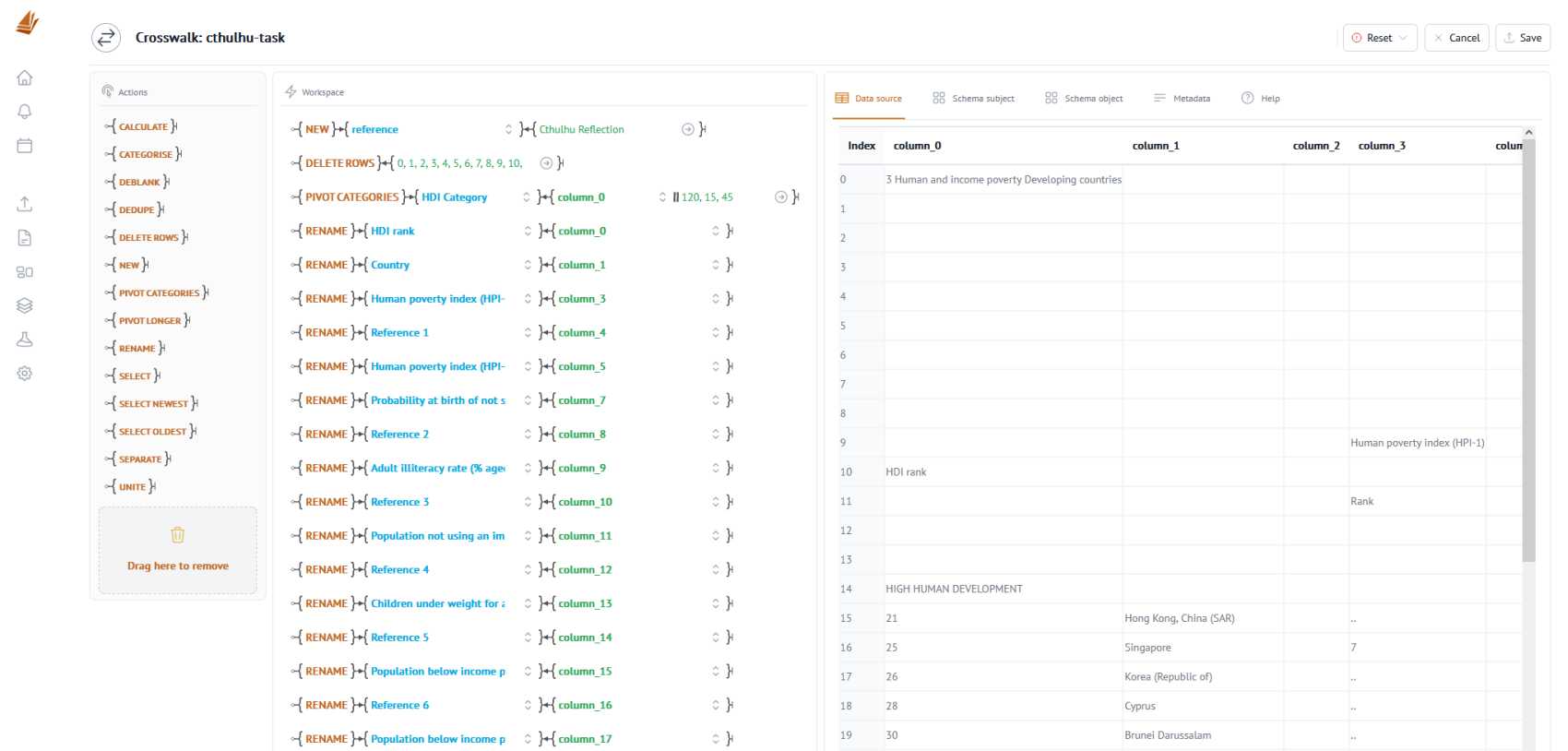
Transform data management
Your data are the foundation for research and decision-support. Ensure interoperability, transparency and probity by using readable, auditable crosswalks.
Derive schemas from source data
Import CSV, XLS or XLSX source files. Derive or coerce unruly data into a defined schema.
![Bring us your ugly data, your mounds of dead files yearning to breathe free. [photo by Aditya at Unsplash]](/img/schemas.jpg)
Define schema-to-schema crosswalks
Drag 'n drop sequential actions to define structured and readable schema-to-schema transform methods.
![Cry neatness, and unleash the methods of transformation. [photo by Nana Smirnova at Unsplash]](/img/transform.jpg)
Manage data science research teams
Manage teams with authentication, and assign rights and tasks. Schedule and track a calendar of data updates and transforms.
![Get thee to the sunny table and let us discurse upon the nature of data management. [photo by Dylan Gillis at Unsplash]](/img/teams.jpg)
Execute and fetch data transforms
Make API calls to bulk create tasks or export transformed data for local automation. Download restructured data as CSV, Excel, Parquet or Feather.
![Send forth the dogs of data discovery and let them retrieve what they find there. [photo by Anthony Duran at Unsplash]](/img/fetch.jpg)
Ensure interoperable standards and validation
Document BibTeX-compliant metadata for projects, collections and source data. Validate output data against an associated transformation method.
![Data swaddled in metadata are like a port in a storm, ensuring safety and onward motion. [photo by Ousa Chea at Unsplash]](/img/interoperable.jpg)
Integrate Whyqd into your workflows
Deploy the open source Whyqd stack as a standalone data science hub on your own infrastructure, or integrated as a Python package in your software.
![Data are tools and the best investment is to ensure their safe custody. [photo by Benjamin Lehman at Unsplash]](/img/integrate.jpg)